Dynamic Knowledge Graphs: Transforming Workforce Skill Analytics
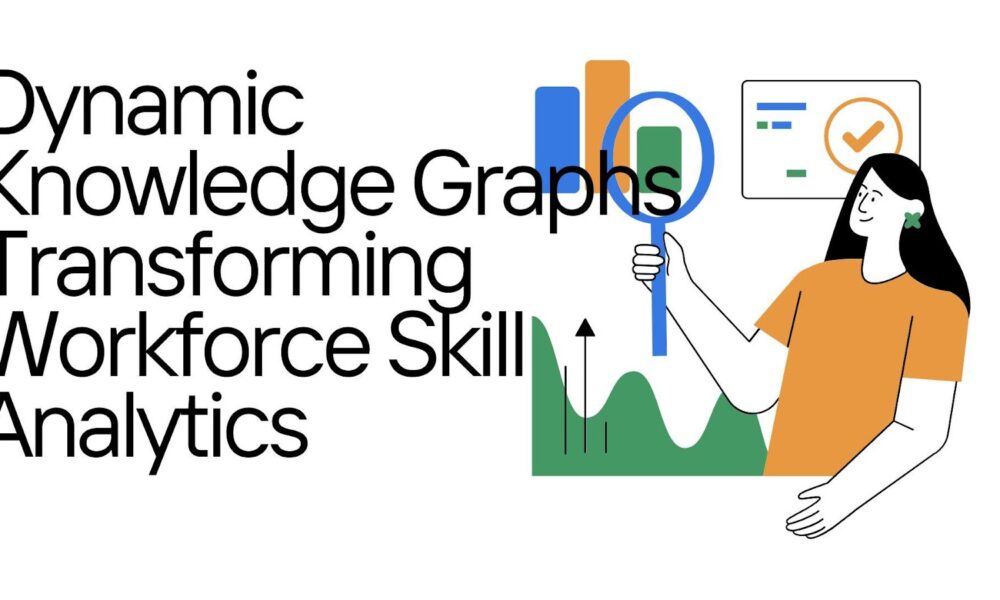
A New Era in Skill Analytics Shekhar Agrawal, along with co-author Rahul Vats, introduces a groundbreaking innovation in workforce skill analytics by leveraging Graph Neural Networks (GNNs). Their research presents a transformative approach to understanding and predicting skill evolution through Dynamic Knowledge Graphs, which hold immense potential for talent management, career planning, and adaptive learning systems.
The Challenge of Workforce Evolution
The modern workforce is undergoing rapid transformation, driven by digital advancements and shifting job roles. Traditional skill management systems struggle to keep pace with these changes, often relying on static knowledge graphs that fail to adapt in real time. The proposed framework addresses this gap by introducing an intelligent system capable of capturing hierarchical skill relationships, predicting emerging trends, and dynamically updating skill taxonomies. Leveraging machine learning and real-time data, this adaptive model enhances workforce planning, upskilling strategies, and talent acquisition, ensuring organizations remain agile in an evolving job market while providing personalized career development insights for employees.
The Power of Graph Neural Networks
At the core of this innovation is a hierarchical Graph Neural Network (GNN) model. Unlike conventional methods, this model efficiently maps parent-child relationships among skills, enabling accurate classification and clustering. The incorporation of GNN technology allows the system to identify underlying patterns in skill evolution, making it a game-changer for workforce development.
Predicting Skill Trends with Temporal Analysis
One of the most significant advancements in this framework is its ability to incorporate temporal analysis. By tracking skill changes over time, the system can predict future demands and emerging competencies with high precision. This ensures that businesses and educational institutions stay ahead of industry shifts, enabling proactive workforce planning and strategic talent development while fostering continuous learning, adaptability, and innovation to meet evolving market needs effectively.
Automated Taxonomy Generation for Skill Classification
Another major innovation is the implementation of an automated taxonomy generation algorithm. Traditional skill classification requires extensive manual intervention, leading to inefficiencies and outdated data. By leveraging unsupervised clustering and natural language processing, the proposed system dynamically updates knowledge graphs, ensuring accurate, scalable, and up-to-date skill taxonomies for enhanced workforce insights.
Applications Across Industries
The implications of this framework extend across multiple sectors. From HR departments streamlining hiring processes to educational institutions enhancing curriculum relevance, the impact of dynamic knowledge graphs is far-reaching. Businesses can leverage this system to identify skill gaps, tailor training programs, and optimize talent acquisition strategies in real time. Additionally, healthcare organizations can use these graphs to track evolving medical knowledge and improve patient care. Financial institutions may apply them for risk assessment and fraud detection, while governments can enhance policy-making through data-driven insights.
Enhanced Efficiency in Workforce Planning
By reducing the time required for data updates and improving data accessibility, organizations can gain faster insights into workforce dynamics. The research highlights that companies implementing knowledge graphs experience a 50% reduction in data integration costs while achieving significantly improved workforce analytics. Furthermore, these organizations benefit from enhanced decision-making, increased operational efficiency, and more accurate talent forecasting. By integrating real-time data streams, businesses can proactively address workforce challenges, predict future skill demands, and align strategic goals with evolving industry trends, ultimately driving innovation and growth.
Bridging the Skills Gap
As industries continue to evolve, the demand for upskilling and reskilling becomes increasingly critical. The framework’s ability to provide real-time insights into skill trends ensures that professionals remain competitive in the job market. Employees can access personalized learning pathways, making career growth more structured and data-driven.
The Future of AI in Skill Development
The integration of AI-driven analytics with workforce planning is poised to revolutionize human resource management. With AI projected to play a crucial role in future job markets, dynamic knowledge graphs offer a scalable solution for tracking skill obsolescence and adapting to rapid industry transformations.
In conclusion ,Shekhar Agrawal and his co-author emphasize the significance of intelligent workforce analytics in the digital era. By utilizing Graph Neural Networks and real-time data processing, organizations can better manage the complexities of modern workforce planning. This groundbreaking approach redefines talent development, helping both employers and employees stay prepared for the future job market.